
-
ResearcherID and Publons have come together
If you have never had a ResearcherID or Publons account, it is easy to to create one. By having a Publons account, you can make your research more visible with a list of your publications with citation information, your h-index, and the peer reviews and editorial works you have done for journals.
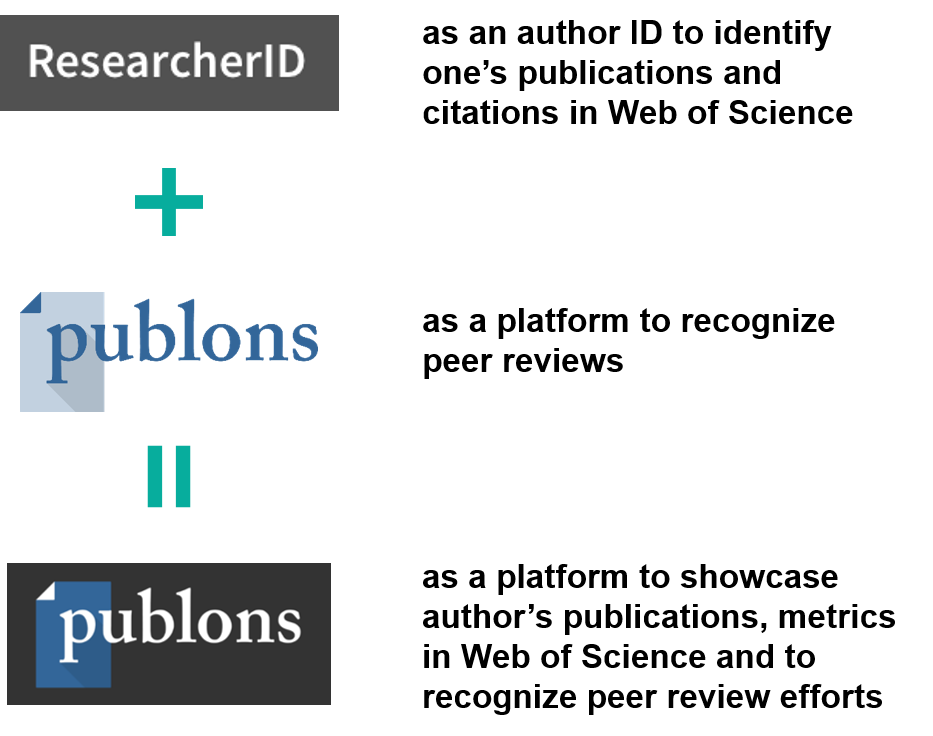
An example of Publons profiles from one of our top reviewers in PolyU. Over 260 PolyU researchers now have an account on Publons.
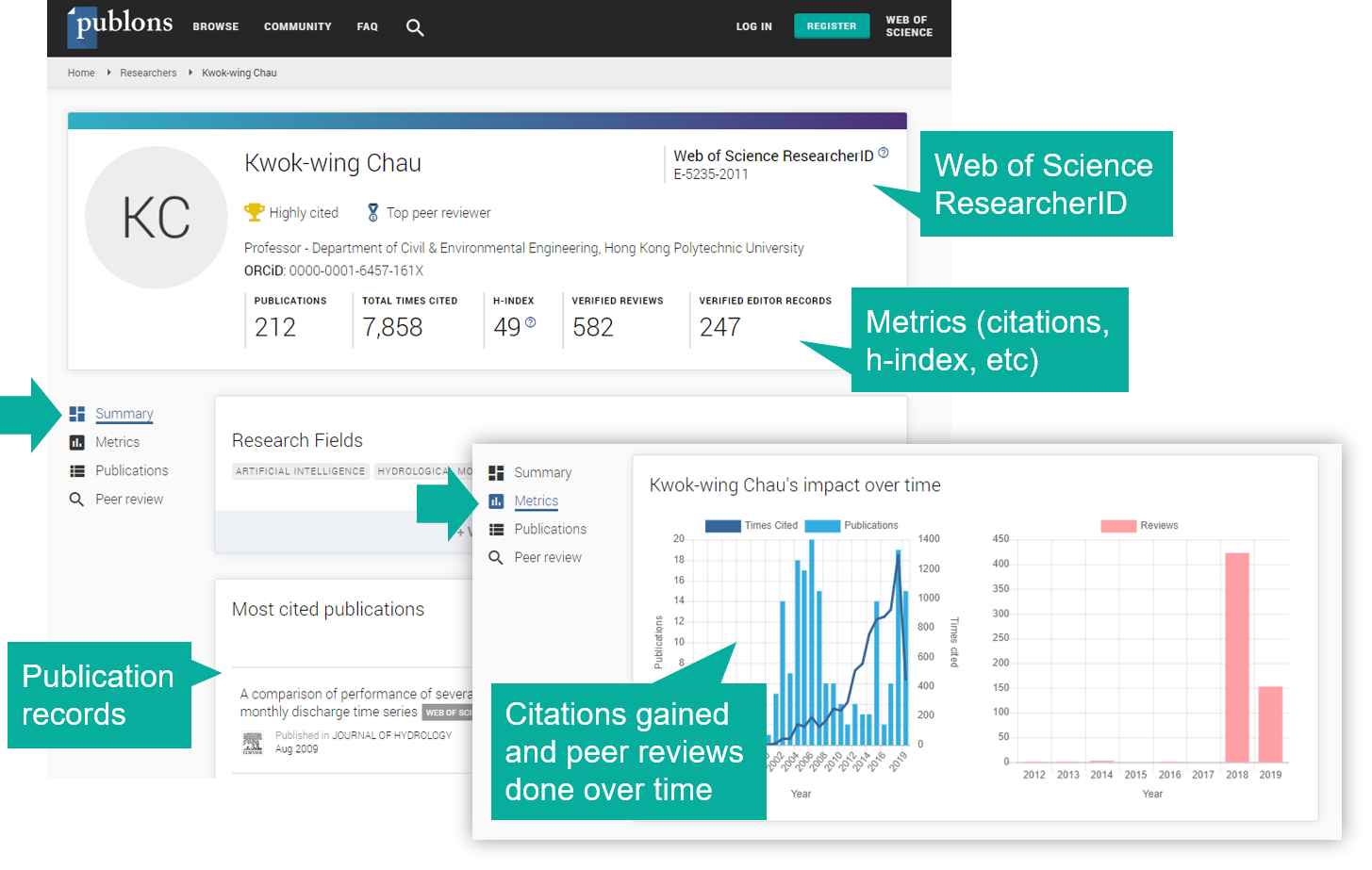
- View all your publications: Old publications on ResearcherID should have been automatically moved to your new Publons account. You can also import your publications from Web of Science or your ORCID account.
- Keep all your peer review records Simply forward “Thank you for reviewing” emails to Publons and they will help you verify the records and add them to your Publons profile. You may also turn on the “Automatically add reviews from partnered journals” in your profile setting and Publons will try to auto-update review records for you.
- Track your citation metrics, including number of publications, total times cited, h-index in Web of Science, and number of reviews done after Publons verified your review records.
- Export academic record that summarizes your scholarly impact as an author, editor and peer reviewer. This could be a useful document with verified information to showcase your research impact and for discussion on your career development.
-
How to find Highly Cited Papers?
Pro tip: Make sure to capture the screen if you hope to keep a proof of your highly cited papers. The data in ESI updates every two months – the top 1% papers do change over time!
How to avoid publishing in “Predatory” journals
As a researcher, apart from losing money, publishing with questionable publishers may harm your credibility and may even get permanently blacklisted from publishing in reputable journals.
![]() |
![]() |
![]() |
![]() |
![]() |
1. Does the journal website look professional? Visit the journal/ publisher’s website. Take a close look at the details, you will probably find poor design, typographical or grammatical errors and even some dead links. |
2. What’s the journal's scope? Does it cover very broad topics? Review the journal’s scope. Most questionable journals have very broad scopes because they will publish articles on any topic. |
3. Who are listed as the member of its editorial board? Check the names of the members of the journal’s editorial board and verify if they are scholars and experts in the field that journal covers. If you are in doubt, contact the members of the editorial board for more information. |
4. Does the journal have peer-review policy? Check the peer-review policy. Reputable publishers provide detailed guidelines for reviewers (An example from a well-known journal - Cell). Predatory journals may not include the peer-review guidelines or they may just include some general information about the peer-review process. |
5. How’s the quality of the published articles in the journal? Examine the articles published in the journal. Check the authors’ affiliations and the quality of the research content. Predatory publishers rarely engage in screening or quality control of the journal articles. |
It is common for “predatory” publishers to make false claims, e.g. the journal has high impact factor, and has been indexed in authoritative citation databases such as Web of Science and Scopus, and persuade authors to submit their articles for publishing. To verify these claims, you may try the following:
- Check Web of Science, Scopus, or other literature indexes/ databases to verify if the journal is indexed.
- Check Journal Citation Reports (JCR) to verify if there is any citation information provided for the journal, such as Journal Impact Factor (JIF) and journal ranking.
- Check Ulrich’s Periodical Directory or other trusted serial directories to verify if the journal has an ISSN, and the publisher’s information.
Visit our guide to learn more about how to select good quality Open Access journals for publishing.
If you are uncertain about whether the journal is trustworthy, feel free to check with your Faculty Librarian for advice.
Tips for data cleaning
Depending on the type of data and the data cleaning tools you select (e.g. Excel, OpenRefine and R), you may need different skills and steps when conducting data cleaning. However, the principles below will always apply when cleaning your data.
![]() |
1. Never work on your raw data directly Data cleaning normally involves a number of steps that may not be easy to undo if a mistake was made during the process. It is a prudent practice to duplicate the raw data and save a back-up before starting to clean it, so that you can always re-work from the raw data. |
![]() |
2. Look at your data before cleaning You should spend time to understand your data before cleaning. Not only look at the column header but also make use of filters or facet tools to preview the data. Are there unique IDs in your data? Are there duplicated records? Is the data consistent? Is there missing data or null value? Can you identify any “outliner”? By doing this, you will be able to identify the data cleaning tasks needed. |
|
3. Avoid manually cleaning Try to avoid manual effort to perform data cleaning. Use available functions and tools instead. This not only saves your time and effort but also avoids the most commonly seen errors introduced by manual input. Learn the functions available in your data cleaning tool can definitely make your life easier. |
|
4. Record your data cleaning steps with explanation It is wise to document every step in your data cleaning process with explanation. This not only allows you replicate the process in the future, but also gives you hints and insights when you need to revisit the previous steps. Proper documentation is beneficial no matter if you are working in a team or working alone. |